The real world benefits of AI: Flamingo case study
Hey! Looks like you have stumbled on the section of our website where we have archived articles from our old business model.
In 2019 the original founding team returned to run Next Investors, we changed our business model to only write about stocks we carefully research and are invested in for the long term.
The below articles were written under our previous business model. We have kept these articles online here for your reference.
Our new mission is to build a high performing ASX micro cap investment portfolio and share our research, analysis and investment strategy with our readers.
Click Here to View Latest Articles
You’ve heard about the growing impact of Artificial Intelligence on the business world, and how it’s set to revolutionise not only how businesses are run but the roles of employees across countless industries.
But have you ever wondered about the specific details of exactly how AI is going to change our lives?
AI and machine learning ASX junior, Flamingo Ai (ASX:FGO) has been making waves in the world of financial services, domestically and internationally, with its ground-breaking virtual assistant software.
Last week, the company released its first Case Study for the use of its machine learning based product MAGGIE — a Cognitive Virtual Assistant for Knowledge Retrieval.
MAGGIE had a two-month trial with International Financial Services Company (FinServCo); one of the world’s largest multinational financial services companies with a presence in over 50 countries and almost 40 million customers.
The problem FinServCo was trying to solve was the fact that employees struggled to efficiently find answers for, and respond to, the following kinds of customer queries: ‘How do I set up an account in multiple regions?’ ‘What forms do I need to fill in and are there different forms for each region?’ and ‘Are there different fees and charges for the account when transacting in different regions?’
The information needed to respond was there, but the company’s knowledge retrieval system struggled to deliver it in a timely manner for these types of questions. Thiscaused lengthy delays in responses to customers and necessitated a process of employees making a time to call the customer back.
This is a classic problem that’s experienced in all sorts of industries where a big company handles customer queries from a centralised contact centre, meaning a lot of employees and many channels through which information must pass.
A complicating factor for FinServCo was that the Customer Service Representative (CSR) was also required to contact a Subject Matter Expert after these types of calls to confirm the correct answer and process, further increasing the time costs for staff.
An inefficient process like this creates problems from several angles. Not only is it inconvenient for the customer, who wants to get the matter resolved in one phone call, it is also time-intensive for several members of staff on each occasion.
Ultimately, the issue could affect the company’s bottom line should the customer get frustrated and take their business elsewhere.
FinServCo estimated that 60% of the Subject Matter Expert’s time was spent following up the request from the CSR to gain more information about the original query and 80% of the requests from CSRs were the same question.This took time away from this staff member’s main task which was to maintain the central documentation containing up-to-date processes for CSR’s — which means the inefficiency was a contributing factor for more negative flow-on effects at the company.
An inefficient knowledge retrieval system can have devastating effects on a large contact centre, leading to immeasurable costs in time and money.
The smart solution: MAGGIE
FinServCo identified a need to change its knowledge retrieval system, and wanted to make sure the solution was as innovative and adaptable as possible. For this, the company required a solution that used AI and machine learning, allowed for scale, could deal with natural language processing, and could be fully automated as well as used in human-machine augmentation mode.
FinServCo engaged FGO and begun a two-month pilot deployment of MAGGIE, the ASX small cap’s Cognitive Virtual Assistant for Knowledge Retrieval.
To test the product out, FinServCo deployed MAGGIE in one region and one department to assist CSRs with query and search processes — and, importantly, help reduce the emails and calls being directed to the Subject Matter Experts (SMEs).
The machine learning process
This is where the Case Study starts to really get interesting. MAGGIE’s ‘brain’ was trained by the company’s SMEs in live mode, and this pre-seeded key information into her machine learning brain.
Next, FinServCo crowd-sourced information from its broader teams in a fun way, and MAGGIE’s brain quickly populated the most frequently asked questions phrased in a variety of ways.
This process took just two weeks — a surprisingly short time period compared to the several months you would expect for a very large company transitioning all its contact centre knowledge from one retrieval system to another. Within just six weeks of signing a contract, MAGGIE was deployed for use by FinServCo.
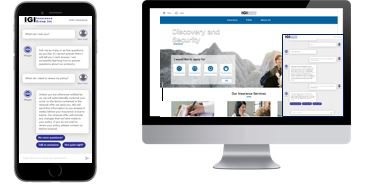
Doing this didn’t just solve the multinational’s original problem of inefficiency, and SMEs being burdened with time-intensive requests, it also had the benefit of finally capturing the important knowledge held by multiple SMEs in a streamlined fashion — and putting it all in a central and accessible place.
What’s really cool from a technology perspective, is that on the few occasions where MAGGIE was not able to provide the correct answer/process, and SMEs were contacted, she learned the new information from then on, eradicating the need to repeat the process the next time.
MAGGIE learned from new questions and answers through a method called unsupervised machine learning and reinforcement learning. When this happens, MAGGIE can only use an answer when it has been reinforced by a human — a simple gatekeeping process where an SME approves the answer.
FinServCo summarises the upside of using AI Assistant MAGGIE
In the Case Study, FinServCo identified some key benefits from using MAGGIE to assist CSRs.
The two most important were an increase in the speed of employees’ responses to customer queries, and a considerable reduction in email requests directed to SMEs. In addition, MAGGIE’s involvement lead to greater compliance in the responses provided to customers, an enhanced ability to keep information up to date with a quick and easy process, and a perhaps unforeseen yet helpful bonus was the fact that MAGGIE was able to be used as a training guide to on-board new employees.
FGO’s Case Study includes a list of further positives of MAGGIE noted during the trial, which include:
- Employees didn’t require training to use the Virtual Assistant as the system was intuitively and very easy to use
- CSRs and employees enjoyed using the Virtual Assistant
- Employees began championing and showcasing the capability to other colleagues across departments
- The FinServCo is looking to use MAGGIE on mobile devices while they are servicing customers
- The ability to use the platform without the need for data scientist teams
- Previous AI projects had taken over six months but FGO delivered within six weeks
With such encouraging results from only a short two-month trial, FinServCo are looking into the option of building on the initial trial and expanding its use into other product lines as well as potentially deploying MAGGIE in more countries.
The company is considering using MAGGIE in future for employee on-boarding and training, as well as the potential for MAGGIE to become a customer facing assistant or ‘web concierge’; using MAGGIE as an afterhours Virtual Assistant in the contact center; and a list of other Use Cases which could service other divisions in the company.
FGO’s other products also gaining interest
Last week, the small cap signed a statement of work with US Fortune 100 company, Nationwide Mutual Insurance, for the use of its machine learning-based analytics and ‘self-organising library’ product, LIBBY.
Nationwide has committed to use LIBBY in one of its departments to analyse large and complex unstructured data-sets. Further, the company will look into LIBBY’s suitability for use across its broader business as an unsupervised machine learning analytics tool.
Nationwide has been a client of FGO’s since 2015, with the relationship formalised in a master services agreement (MSA) with Nationwide since May 2016.
The contract represents FGO’s first paid engagement of LIBBY, with the company noting that it has received strong interest in the product in the US and Asia Pacific from multiple players in the insurance, banking and investment industries.
LIBBY is one of FGO’s newest products, whose core capability is the automatic structuring of unstructured data. To do this, LIBBY utilises IP that FGO built entirely in-house.
The application solves a key business problem – the inefficiency of enterprises to manage, store, retrieve, analyse and gain insights from their vast quantities of unstructured, conversational and non-form data.
Share price climbs following FGO’s string of positive news
After a tough year for FGO along with many other ASX small caps, the AI tech plays recent positive news — including this Case Study which was published on its website last week — has seen it recover an impressive ~58% since the beginning of last week.
General Information Only
S3 Consortium Pty Ltd (S3, ‘we’, ‘us’, ‘our’) (CAR No. 433913) is a corporate authorised representative of LeMessurier Securities Pty Ltd (AFSL No. 296877). The information contained in this article is general information and is for informational purposes only. Any advice is general advice only. Any advice contained in this article does not constitute personal advice and S3 has not taken into consideration your personal objectives, financial situation or needs. Please seek your own independent professional advice before making any financial investment decision. Those persons acting upon information contained in this article do so entirely at their own risk.
Conflicts of Interest Notice
S3 and its associated entities may hold investments in companies featured in its articles, including through being paid in the securities of the companies we provide commentary on. We disclose the securities held in relation to a particular company that we provide commentary on. Refer to our Disclosure Policy for information on our self-imposed trading blackouts, hold conditions and de-risking (sell conditions) which seek to mitigate against any potential conflicts of interest.
Publication Notice and Disclaimer
The information contained in this article is current as at the publication date. At the time of publishing, the information contained in this article is based on sources which are available in the public domain that we consider to be reliable, and our own analysis of those sources. The views of the author may not reflect the views of the AFSL holder. Any decision by you to purchase securities in the companies featured in this article should be done so after you have sought your own independent professional advice regarding this information and made your own inquiries as to the validity of any information in this article.
Any forward-looking statements contained in this article are not guarantees or predictions of future performance, and involve known and unknown risks, uncertainties and other factors, many of which are beyond our control, and which may cause actual results or performance of companies featured to differ materially from those expressed in the statements contained in this article. S3 cannot and does not give any assurance that the results or performance expressed or implied by any forward-looking statements contained in this article will actually occur and readers are cautioned not to put undue reliance on forward-looking statements.
This article may include references to our past investing performance. Past performance is not a reliable indicator of our future investing performance.